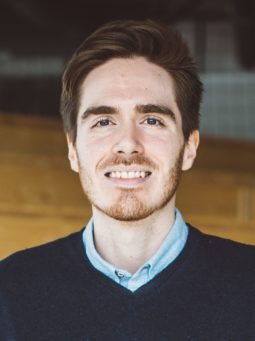
Santiago de Leon
Research areas: eye tracking and recommender systems; psychiatric machine learning
Position: PhD Student MSCA Doctoral Network
I am a Spanish American PhD student at KInIT in the MSCA Eyes4ICU doctoral network. I have a master’s degree in Health Information Engineering from the University Carlos III of Madrid, with previous research experience in physics, neurobiology, chemistry, and mathematics.
I began university level research in high school, as I was selected for a gifted and talented program. At my undergraduate institution, the University of Kentucky, I received the highest merit-based scholarship and graduated with three bachelor degrees in 4 years: mathematics, chemistry, and Spanish.
In 2018, I joined two ongoing research groups in Madrid, Spain, one in psychiatry at the Jiménez Díaz Foundation and the other in machine learning at the University Carlos III of Madrid.
I have multiple publications spanning the fields of mathematical number theory, psychiatry, and machine learning. My current research is focused on the evaluation of users and recommender systems through eye tracking analysis.
PhD topic: Eye-tracking the consumption of recommended information online
Supervising team: Mária Bieliková (KInIT), Róbert Móro (KInIT)
Objectives: Recommendation uses machine learning to predict users’ interest in the content from the users’ interaction, mostly clicks. However, there is a presentation bias (users tend to click more on the first items in the list) and self-selection bias (users tend to rate more items they like than the ones they dislike) in play in the observed data which negatively impacts the performance of the recommender systems. The objective of the project is to use remote eye tracking to:
(1) model the users’ consumption of the recommended items and their interaction with the recommender systems,
(2) debias the observed implicit feedback and
(3) improve existing offline and online evaluation methodologies.
From the eye tracking perspective, the challenge lies in the dynamic nature of the web interfaces where the recommendations are presented; content (targets) can change position over time or they can be overlayed by a different content; the project will therefore also contribute to the development of methods for mapping gaze to targets, while also accounting for the inaccuracies in the measurements that can arise from using eye tracking in an uncontrolled environment of the web.
Expected Results:
1) Computational model of users’ interaction with the recommender systems explicitly modeling positional, visual, and other biases by means of eye tracking;
(2) Evaluation methodology for recommender systems incorporating remote eye tracking;
(3) Computational methods of the collected gaze data processing adapted for the dynamic nature of the web focusing on mapping the gaze to the correct targets (elements/items on the webpage) and accounting for possible inaccuracies in the measurements