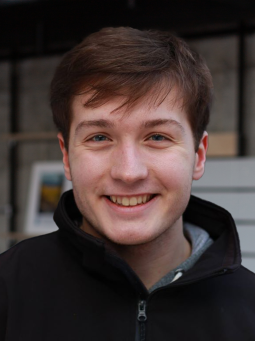
Róbert Belanec
Research areas: Machine learning with limited (labelled) data
Position: PhD Student
Róbert Belanec is a PhD student focusing on solving tasks such as disinformation spreading, automated fact-checking or auditing, and analyzing the capabilities of popular big language models with the utilization of methods of machine learning with limited data together with methods of natural language processing. He is part of the Web & User Data Processing team.
He holds a master’s degree from Faculty of Mathematics, Physics and Informatics, Comenius University Bratislava, where he graduated with honors. Both of his bachelor’s (Realistic image synthesis, based on a description in natural language) and master’s (Controlling the output of a generative model by finding latent feature representations) theses were related to generative models (mostly GANs for image generation) and the methods of controlling their output without further training.
During his studies he also gained three years of knowledge and experience from DevOPS and operating systems in Slovak web hosting company (Websupport).
Nowadays, there are many problems that we can solve by using machine learning methods and algorithms. However, even the most potent methods are often limited to the available data. If available, supervised learning methods often require the data to be labeled, which is not that common in real situations, and label acquisition is often financially expensive. Therefore, a necessity arises for other machine learning methods like transfer-learning, meta-learning, or zero/one-shot learning to be developed.
Machine learning methods with limited data are especially important in domains that constantly lack labeled data (e.g., due to never-ending significant concept and data drifts), such as disinformation detection, hate speech detection, or fact-checking. These domains are recently attracting considerable attention from researchers in computer science and particularly in machine learning and natural language processing. With the increasing social media trend, people are often exposed to a vast amount of information within a short period of time. This information is usually not factual or proven, and it’s upon the recipient to construct an opinion. Sometimes the information may be harmful, like hate speech, or it may be disinformation. To prevent the spread of this kind of information, we can train a language model that can detect disinformation or harmful information (i.e., disinformation may often be written deontic flavor).
Róbert’s orientation in this topic will point towards the utilization of methods of machine learning with limited data together with methods of natural language processing to solve tasks such as disinformation spreading, automated fact-checking or auditing, and analyzing the capabilities of popular big language models for this purpose.
News
- [June 2024] Preprint of my paper entitled “Task Prompt Vectors: Effective Initialization through Multi-Task Soft-Prompt Transfer” is now available online
- [March 2024] I finalized a technical report from my replication study: “ATTEMPT – Parameter-Efficient Multi-task Tuning via Attentional Mixtures of Soft Prompts: A Replication Study“